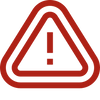
The Challenge
Health-Checks:
Designers are unable to monitor their designs after launch besides depending on ambiguous business KPIs (e.g. NPS, LTR, CSAT, etc.). This makes it challenging to determine how their designs actually perform in the “wild” (i.e. health-check).
In turn, it makes it challenging for Designers to understand customer behavior on their UIs, and how this behavior compares against the intended design.
Designer-to-researcher ratio:
A team of 50+ Designers are primarily dependent on 3 Researchers to make informed iterations.
How do we equip Designers with data tools to make their own quick design iterations? i.e. How do we scale our research?
Business and Design don't meet eye-to-eye:
UX designers can still be perceived as “pixel pushers” by traditional business units. Hence, these business units (e.g. brand, marketing, etc.) use analytics to dictate how designs should look like.
In a lot of the cases, these analytics are usually vanity metrics (e.g. raw page views, time spent on page, etc.) which speak little to how a user experiences a UI
UX Designers have scarce resources/tools to have meaningful conversations about analytics and behaviours, and how these can be compromised by the business unit.
Health-Checks:
Designers are unable to monitor their designs after launch besides depending on ambiguous business KPIs (e.g. NPS, LTR, CSAT, etc.). This makes it challenging to determine how their designs actually perform in the “wild” (i.e. health-check).
In turn, it makes it challenging for Designers to understand customer behavior on their UIs, and how this behavior compares against the intended design.
Designer-to-researcher ratio:
A team of 50+ Designers are primarily dependent on 3 Researchers to make informed iterations.
How do we equip Designers with data tools to make their own quick design iterations? i.e. How do we scale our research?
Business and Design don't meet eye-to-eye:
UX designers can still be perceived as “pixel pushers” by traditional business units. Hence, these business units (e.g. brand, marketing, etc.) use analytics to dictate how designs should look like.
In a lot of the cases, these analytics are usually vanity metrics (e.g. raw page views, time spent on page, etc.) which speak little to how a user experiences a UI
UX Designers have scarce resources/tools to have meaningful conversations about analytics and behaviours, and how these can be compromised by the business unit.

The Solution
G.A.M.E. Framework + Google’s H.E.A.R.T. Framework:
Google’s HEART framework assumes that a company is already centrally functioning through a UX capacity (i.e. the business goal and UX goals are identical). This is not the case for traditional companies.
The GAME framework is more relevant for traditional companies instead. It helps translate the business goal into a UX goal which can be further broken down into actions and metrics.
These two frameworks can be combined at any capacity towards any company irrespective of the state of UX.
G.A.M.E. Framework + Google’s H.E.A.R.T. Framework:
Google’s HEART framework assumes that a company is already centrally functioning through a UX capacity (i.e. the business goal and UX goals are identical). This is not the case for traditional companies.
The GAME framework is more relevant for traditional companies instead. It helps translate the business goal into a UX goal which can be further broken down into actions and metrics.
These two frameworks can be combined at any capacity towards any company irrespective of the state of UX.
G.A.M.E. Framework
Goal:
Start high level (top-down).
Identify business and UX goals of the product/page/feature.
Action:
Outline user actions/behaviours required to meet UX and business goals.
Actions/behaviors should be chosen within the categories of the HEART framework.
Metric:
Quantify actions (behavioural data that need to be tracked over time).
Evaluate:
Evaluate designs.
Google's H.E.A.R.T. Framework
Happiness:
The measure of user attitude (e.g. satisfaction, perceived ease of use, SUS, NPS).
Engagement:
The measure of user involvement (e.g. frequency, intensity, depth of interaction overtime period).
Adoption:
The measure of new users of a product or feature (e.g. # of accounts created, % of users that use a feature).
Retention:
The measure of the rate of existing users returning (e.g. # of active users returning with a time period, churn).
Task Success:
The traditional behavioral metrics of user experience (e.g. time to complete a task, % of tasks completed, error rate).
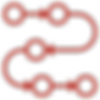
A Case Study
Let's look at a Smart Home Monitoring product sales page:
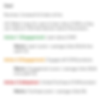

Results:

Derived from Clicktale (Note: These data points are theoretical and only used to illustrate the content of this page).
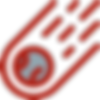
The Impact
The Product Team can now see a detailed breakdown of how users behave on this page.
Based on the vertical bar graph, users spend little time on Action 1 (Learning about SHM) but spend more time on Action 2 (Engaging with SHM products) and Action 3 (Initiating the purchase journey) instead.
At surface level, this looks good as users are spending an ideal amount of time on Action 2 and Action 3. Though, Designers now know that users are not learning about the concept of SHM before moving forward with the flow (i.e. they are not informing themselves). Hence, more users drop off when purchasing SHM as they haven’t fully adopted the product yet.
Designers can now expand on each Action (horizontal bar graph) and determine which component to improve towards the intended behavior.